IDENTIFICATION AND SELECTION OF THE DESCRIPTORS FOR ESTABLISHING A SENSORY PROFILE OF TOMATO BY A MULTIDIMENSIONAL APPROACH
ABSTRACT
Introduction
MATERIAL AND METHODS
Samples
Sensory evaluation
Data analysis
Results and Discussion
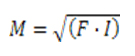
where is:
F – number of descriptor’s mentions divided by the total possible number of this descriptor’s mentions, expressed as percentage.
I – sum of intensities given by the entire panel for descriptor divided by the maximum possible intensity of this descriptor, expressed as percentage.
Descriptor |
Abbreviation |
M – value (%) |
||||
Product 1 |
Product 2 |
Product 3 |
Product 4 |
Product 5 |
||
Whole fruit |
|
|
|
|
|
|
Shape regularity |
SH |
81.24 |
84.85 |
88.32 |
70.71 |
73.48 |
Size |
S |
63.89 |
60.61 |
47.38 |
53.45 |
49.49 |
Colour |
C |
82.81 |
95.62 |
87.83 |
67.61 |
65.47 |
Colour uniformity |
CU |
81.06 |
94.11 |
91.03 |
58.55 |
58.55 |
Surface smoothness |
SS |
25.56 |
23.90 |
23.90 |
22.13 |
20.20 |
Mechanical damage |
MD |
43.89 |
49.19 |
32.20 |
51.64 |
51.64 |
Scar size |
SCS |
12.78 |
11.07 |
9.04 |
11.07 |
9.04 |
Scar regularity |
SCR |
6.39 |
12.78 |
14.29 |
6.39 |
11.07 |
Skin cleanness |
SC |
23.90 |
23.90 |
25.56 |
18.07 |
18.07 |
Skin brightness |
SB |
60.61 |
49.49 |
60.61 |
47.38 |
47.38 |
Surface green decolourization |
SGD |
15.65 |
20.20 |
20.20 |
27.11 |
9.04 |
Firmness |
F |
83.00 |
89.44 |
85.63 |
68.31 |
84.33 |
Skin wilting |
SW |
42.86 |
45.18 |
42.86 |
49.49 |
53.45 |
Skin elasticity |
SE |
15.65 |
11.07 |
22.13 |
27.11 |
25.56 |
Cross -section |
|
|
|
|
|
|
Cross-section colour |
CSC |
47.38 |
62.27 |
63.89 |
55.33 |
62.27 |
Cross-section colour uniformity |
CSCU |
68.21 |
63.89 |
73.40 |
58.55 |
73.40 |
Fruit fleshiness |
FF |
22.13 |
22.13 |
22.13 |
28.57 |
27.11 |
Fruit compactness |
FC |
58.90 |
60.61 |
49.49 |
67.01 |
60.61 |
Juice leakage |
JL |
57.14 |
55.33 |
62.27 |
60.61 |
64.52 |
Vessels |
V |
58.55 |
58.55 |
77.46 |
65.47 |
73.68 |
Unripe layers |
UL |
12.78 |
9.04 |
14.29 |
6.39 |
9.04 |
Cross-section green decolourization |
CSGD |
6.39 |
6.39 |
6.39 |
12.78 |
9.04 |
Skin thickness |
ST |
22.13 |
22.13 |
18.07 |
25.56 |
23.90 |
Skin peeling |
SP |
33.20 |
33.20 |
15.65 |
15.65 |
15.65 |
Seeds |
SEEDS |
12.78 |
12.78 |
11.07 |
6.39 |
9.04 |
Texture in mouth |
|
|
|
|
|
|
Skin chewiness |
SCH |
49.49 |
62.60 |
71.71 |
71.71 |
47.81 |
Firmness |
FM |
40.41 |
34.99 |
38.33 |
27.11 |
31.30 |
Solubility |
SM |
11.07 |
12.78 |
9.04 |
14.29 |
14.29 |
Juiciness |
J |
33.20 |
46.07 |
47.81 |
44.26 |
33.20 |
Chewiness |
CH |
53.45 |
69.99 |
53.45 |
75.05 |
51.11 |
Mealiness |
M |
46.07 |
31.30 |
29.28 |
19.17 |
24.74 |
Covering of oral cavity |
COC |
12.78 |
11.07 |
12.78 |
12.78 |
12.78 |
Odour and taste |
|
|
|
|
|
|
Odour |
OD |
84.52 |
79.28 |
69.69 |
56.42 |
63.25 |
Off-odour |
OOD |
6.39 |
6.39 |
6.39 |
18.07 |
9.04 |
Sour taste |
SOT |
44.26 |
36.14 |
49.49 |
42.38 |
36.14 |
Sweet taste |
SWT |
56.42 |
51.51 |
77.46 |
53.45 |
71.71 |
Off-taste |
OFFT |
12.78 |
9.04 |
14.29 |
6.39 |
9.04 |
Flavour |
FL |
15.65 |
22.13 |
22.13 |
20.20 |
27.11 |
After taste |
AT |
33.20 |
34.99 |
20.20 |
25.56 |
38.33 |
Descriptor |
F1 |
F2 |
SH |
0.864 |
0.000 |
S |
0.023 |
0.966 |
C |
0.580 |
0.097 |
CU |
0.715 |
0.039 |
MD |
0.643 |
0.199 |
SCR |
0.272 |
0.255 |
SB |
0.703 |
0.001 |
F |
0.604 |
0.008 |
CSC |
0.006 |
0.413 |
CSCU |
0.382 |
0.246 |
FC |
0.774 |
0.225 |
JL |
0.067 |
0.739 |
V |
0.004 |
0.966 |
SP |
0.173 |
0.810 |
SCH |
0.010 |
0.157 |
FM |
0.912 |
0.043 |
J |
0.009 |
0.092 |
CH |
0.346 |
0.099 |
M |
0.503 |
0.347 |
OD |
0.577 |
0.417 |
OOD |
0.825 |
0.017 |
SOT |
0.207 |
0.157 |
SWT |
0.140 |
0.740 |
OFFT |
0.868 |
0.038 |
AT |
0.026 |
0.298 |
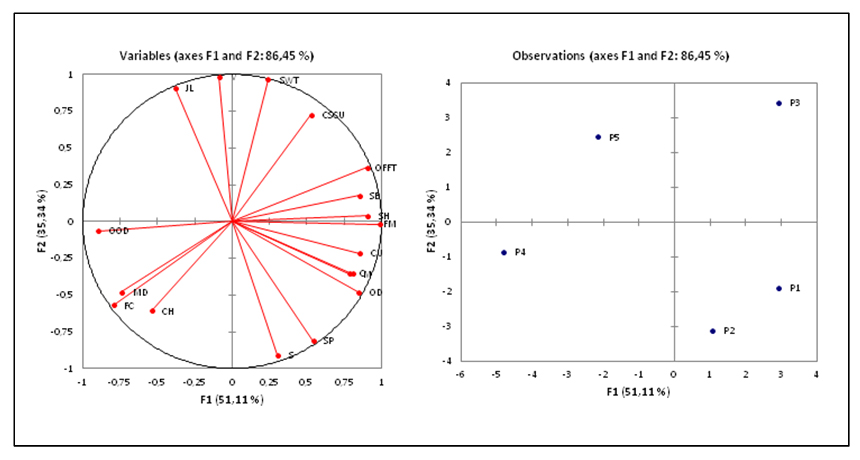
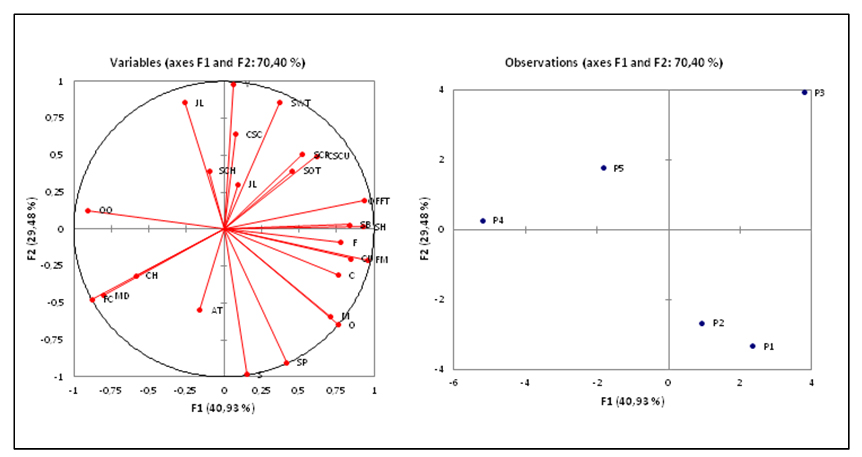
Established sensory profile of fresh tomato is presented in Table 3.
Descriptor |
Abbreviation |
Definition |
APPEARANCE |
||
Whole fruit |
||
Shape regularity |
SH |
Symmetry of the fruit (cultivar characteristic) |
Size |
S |
Size of the fruit (cultivar characteristic) |
Colour |
C |
Intensity of fruit red colour |
Colour uniformity |
CU |
Areas coloured different from red |
Mechanical damage |
MD |
Size and number of scars and bruises |
Skin brightness |
SB |
Reflection of light from skin |
Cross-section of the fruit |
||
Cross-section colour uniformity |
CSCU |
Areas coloured different from red in the cross-section |
Fruit compactness |
FC |
Appearance of cavities in the cross-section |
Juice leakage |
JL |
Amount of juice leaked after cutting with knife |
Vessels |
V |
Number of vessels seen in the cross-section |
Skin peeling |
SP |
Amount of peel separated from mesocarp after cutting by knife |
TEXTURE IN MOUTH |
||
Firmness |
FM |
The force required to cut through the tomato sample using the front teeth. |
Chewiness |
CH |
The length of time required to masticate the tomato to a state of swallowing. |
Mealiness |
M |
Geometrical texture attribute relating to the perception of the size and shape of particle in the tomato sample |
ODOUR |
||
Odour |
OD |
Tomato characteristic odour |
Off-odour |
OOD |
Non characteristic odour |
TASTE |
||
Sweet taste |
ST |
The fundamental taste associated with a sucrose solution |
Off-taste |
OFFT |
Non characteristic taste |
Conclusions
This research showed that the application of the multidimensional method can be suitable tool for identifying and selecting descriptors for establishing the sensory profile of the fresh tomato. These descriptors are useful for quality differentiation of tomatoes on the market, and determination of the optimal harvest maturity and storage conditions.ACKNOWLEDGEMENTS
This paper is a result of the research within the project III 46001, financed by the Ministry of Education and Science, Republic of Serbia.